Professor David Clifton leads the Computational Health Informatics (CHI) Lab within the Institute of Biomedical Engineering at the University of Oxford. He was recently appointed to the GlaxoSmithKline/Royal Academy of Engineering Chair in Clinical Machine Learning and an NIHR Research Professorship. Here he discusses how he strikes the right balance between AI theory and AI practice to build real tools for social good.
What is the focus of these new roles?
These represent both halves of my research life: the Royal Academy of Engineering Chair is focused on developing Medical AI theory – the Royal Academy is the national academy for our discipline, and their goal is ensuring that the UK maintains and extends its competitive advantages in all branches of engineering, including AI. That programme is joint-sponsored by one of the UK’s largest companies, GlaxoSmithKline, which has AI as one of its core interests for developing new medicines.
The NIHR, on the other hand, is the research branch of the NHS, and which gives over £1.2 billion to UK medical research each year. Their concern is improving medical care, and so the focus of that scheme is on delivering novel AI-based technologies into the NHS.
Is there difficulty in striking a balance between the two?
I think the real “secret sauce”, certainly in Medical AI, is striking that balance between AI theory and AI practice.
There is a ton of exciting stuff to do in the new era of Medical AI – from massive-scale foundational models to careful statistical modelling. Lots of the young researchers who join our Oxford team are attracted by getting the papers into the “right” AI conferences, which is what advances most people’s AI careers, and Oxford is rightly seen as one of the world’s hotspots for AI training.
However, what our Department also does really well is that, once people have joined, they become part of projects that are building real tools for social good. In my case, that’s putting things into the hands of medics to help real patients. There’s something truly exciting about using AI to make a positive difference to the world beyond academic papers, and Oxford has one of the world’s largest and most successful medical schools that has an unquenchable need for AI, given the size and complexity of data that are now routinely available in healthcare.
Give us some examples of what this kind of research means to you.
The kind of AI my team specialises in is things like large language models for medicine, sensor data (from devices like wearables), genetic data, hospital records, and so on. Anything that’s not an image, basically – there’s a lot of great people that already do imaging, particularly here at Oxford. So, a real emphasis of the work is trying to make sense of data that we get from literally millions of patients – the scale has really changed within the last few years. You need new AI methods to handle those quantities of data – you can’t just turn up with your grandma’s favourite neural network toolbox from the 1990s!
The new scale unlocks lots of new capabilities that were previously not imaginable; for example, a recent creation from our team is “DrugGPT”, a system for making accurate recommendations for using medicines, without the “hallucination” errors of generic models like ChatGPT, which are trained on huge amounts of general text. Within healthcare, there is a relatively controlled vocabulary, and so we can exploit that by building safe AI systems that have lower error rates than humans when it comes to, for example, trying to identify safe combinations of drugs to prescribe to a patient.
There’s much written about the threat of AI – is there a threat in healthcare that it will replace humans?
No-one is going to want a “computer says no” problem in hospital - we need the human expert to make the final decision. However, AI can really help humans. The third biggest killer in the US last year was not a disease – it was medical error. This makes sense when you think about how complex healthcare is, how busy hospitals can be, and how patients vary so greatly. So, I think a big role for AI is catching and fixing human errors. For example, you get a letter to take home when you leave hospital, which tells you what you should be doing (and which medicines you should be taking) – there is plenty of scope for human error in this scenario, because the person writing the letters needs to examine the entire patient’s history and their hospital records.
One tool from our lab effectively provides a safe, low-error “first draft” of this letter, which a human can then modify as necessary before approving it – rather than the human having to try and create it all from scratch. I don’t know if you’ve ever read a doctor’s letter, but they’re often not Shakespearian standard! We can match their fluency in writing, while catching a lot of errors that might otherwise creep in. This means fewer problems for patients, and freeing up the human from what would otherwise be quite a time-consuming task. AI is great in these “co-pilot” settings.
With interest in AI exploding across the world, is it difficult to get scientists to join a university research lab?
That’s a great question – the times have really changed. A few years ago, we’d get graduate students who’d be coming to get their PhD on the way to working for the big tech companies. That’s still a great destination for our graduates, but we’re also now seeing a big surge of really great scientists who are coming into academic careers because of the job stability, compared to the well-documented turbulence of many large tech firms. Also, especially in Medical AI, people are seeing that it’s a hot new area – and one that is actually pretty hard to do anywhere except universities because you need huge datasets, clinicians to work with you to explain the data, and to get all of the complex ethics and governance approvals in place. Modern universities – and Oxford in particular – make it really easy to build an exciting academic career while getting involved with industry, spin-outs, and so on.
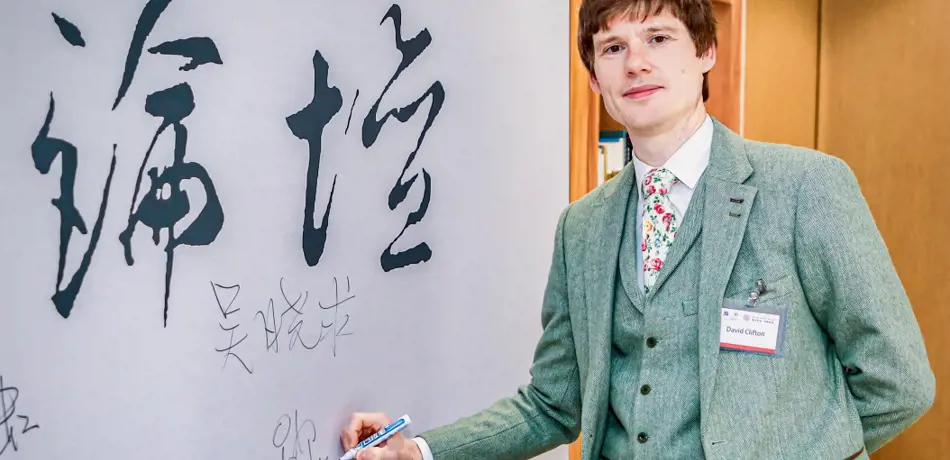
Has your field changed as a result of all of the developments in AI?
A few things are the same as when I was a postdoc nine years ago – you still need to study the mathematical basis if you’re going to make a career in the field. However, AI can also be really accessible – my kids are being taught it at school in fact – but that’s the easier “commodity” end of the field. You can teach them to run a toolbox and to call functions from a pre-created library, but you’re going to need to put in the hours (ideally at a university lab!) to work on the new generation of technologies and really understand what’s going on “under the bonnet”.
What has really changed is the average medical researcher’s opinion of AI. In my postdoc years, I remember having to “set out the stall” to show what AI can do for medics. These days, with AI being a big part of everyone’s everyday life, it’s the other way around: medical scientists are now coming to us, with huge datasets and a problem they want to solve. They work with us to build something that can solve the problem. We’re very lucky to have such a good environment as we have here at Oxford to do this kind of work – it’s not very common globally, because millions of patients of health data isn’t something you can download from a web-site. Plus, we want to work with the health tech industry, like my RAEng Chair sponsors, GSK, to build something that gets used in practice. You’ve got nothing to fear from Medical AI!
Why did you get into AI in the first place?
I like to solve problems, and maybe it runs in the family. My ancestors came to England in the 1500s, when Queen Elizabeth I invited ten families of engineers (“and their retainers”) from the continent to reclaim East Anglia from the sea. The problems of their day were around building structures that we still use in the region today to control floods, keep water flowing around fields for irrigation, and so on. There’s even a university out there in the Fenland. If those engineers were around today, I like to think that they might be hacking away at a Python script instead of building windmill-style waterpumps. Did you always want to be a scientist? It was a close call, as I was thinking about being a priest, believe it or not. One of my family was a Doctor of Divinity, from Keble College here in Oxford. The apple never falls far from the tree…