Cryogenics Fluid Dynamics Lab, Department of Engineering Science, University of Oxford: Machine Learning Approaches Tailored for Cryogenic Fluid Dynamics Predictions
Machine Learning Approaches For Cryogenic Fluids
Machine Learning Approaches Tailored for Cryogenic Fluid Dynamics Predictions
Machine learning (ML) can significantly enhance computational fluid dynamics (CFD) simulations of cryogenic fluids by improving the accuracy and efficiency of predictive models. The ML algorithms we develop within the group focus on
- Automating the categorisation of fluid behaviours, by training the algorithms on simulation data to learn complex relationships between input parameters and fluid behaviour.
- Enabling the development of more accurate turbulence models and fluid property correlations specific to cryogenic conditions.
- Optimising mesh generation, reducing computational overhead, and guiding adaptive refinement strategies.
By integrating ML techniques into CFD simulations of cryogenic fluids, we gain deeper insights into fluid dynamics, optimize system designs, and enhance efficiency in cryogenic applications such as storage, rocket propulsion, and superconducting technologies.
In applications involving cryogenic fuels such as hydrogen and liquefied natural gas (LNG), ML offers innovative solutions to enhance safety assessments. Currently, in our lab, we utilize ML regression models to predict critical parameters like temperature, pressure, and flow rates within cryogenic systems. These models are trained on historical data as well as synthetic data generated through our own CFD tools. By analysing past operational data from hydrogen storage tanks, for example, regression models can identify abnormal conditions indicative of potential leaks or malfunctions, thus enhancing safety measures. Additionally, ML-based time-series forecasting allows us to predict future trends and behaviour patterns in cryogenic fluid dynamics. By analysing temporal data sequences, these models can anticipate system responses to varying operational conditions and external factors, aiding in proactive safety planning. For instance, time-series forecasting can predict hydrogen consumption rates in fuel cells or anticipate pressure fluctuations in cryogenic pipelines, enabling timely interventions to prevent potential safety hazards.
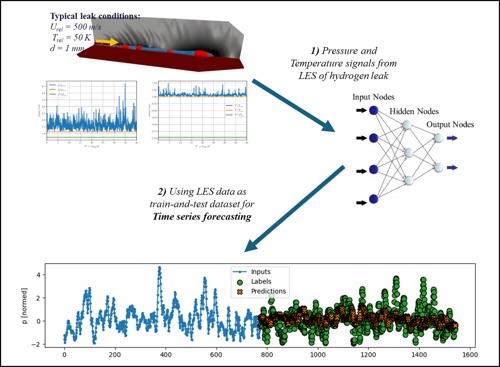