PULSE (Ultrasound) Research at the Engineering Science Department - University of Oxford
Research
Our research has three main strands
Research Collage
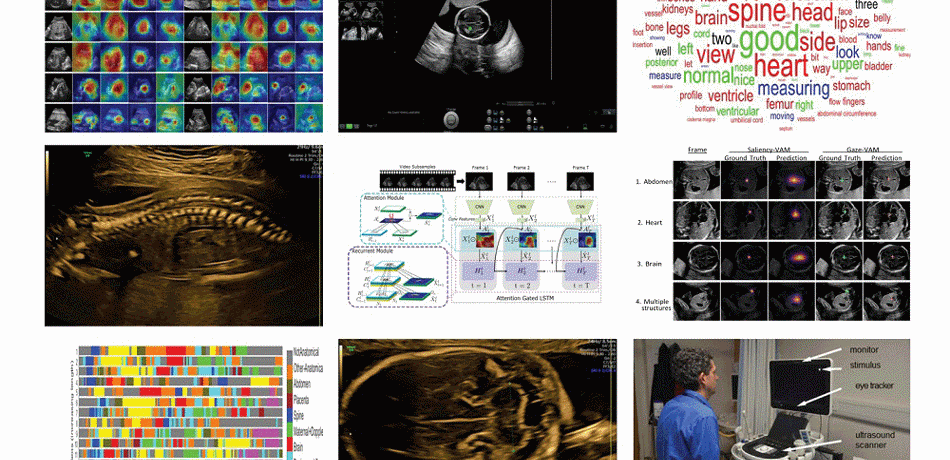
Watch our video showing the sonographer's gaze (the green dot) during a scan
Watch our video showing the sonographer's gaze (the green dot) during a scan
Describing ultrasound video content
Our aim is to build very rich computational descriptions of ultrasound video content using deep learning architectures that are potentially useful for clinical applications such as diagnosis, information recall, training and audit.
Our current research includes:
- Unsupervised ultrasound image representation learning.
- Design of efficient spatio-temporal CNN architectures for ultrasound video.
- Hierarchical life-long learning for image representation learning.
- Dynamic neural attention models.
- Automatic captioning of ultrasound video.
Spatio-temporal partitioning and classification of full-length fetal anomaly scan videos
Spatio-temporal partitioning and classification of full-length fetal anomaly scan videos
'Big data' sonography
Our aim is to enhance understanding of human visual search and navigation in clinical sonography by large-scale data analysis of simultaneously acquired ultrasound, eye-tracking and probe motion data.
We are collecting a large dataset of 1000 full obstetric ultrasound scans with sonographer eye-movement and probe motion data simultaneously acquired for this purpose. This amounts to about 750 hours of clinical workflow/skills assessment video.
We are studying the human visual search and navigation strategies employee by highly-trained sonographers in task-oriented scenarios.
We are studying whether minimally-trained users and experts follow different search strategies, and whether there are different strategies amongst experts.
Knowledge gleaned will lead to better understanding of skills assessment, clinical workflow optimisation and assistive device design.