SAGEflex: Safeguarded AI Agents for Grid-Edge Flexibility
Dates
Start: 27 Feb 2025
End: 26 May 2026
Project Description
The transition to net-zero carbon requires integrating millions of grid-edge devices (electric vehicles, heat pumps, batteries) into the Great Britain power system over the next decade. These devices can provide flexibility equivalent to multiple large power plants, but traditional centralised dispatch is infeasible due to computational and communication challenges.
The International Energy Agency’s 3DEN initiative estimates smart flexibility could reduce global network reinforcement costs by USD $270bn to 2040 (link: www.iea.org/reports/digitalisation-and-energy). Multi-Agent Reinforcement Learning (MARL) offers a scalable AI-based coordination solution. However, MARL lacks safety guarantees, preventing industrial adoption by system operators and flexibility aggregators.
At the same time, the Advanced Research + Invention Agency (ARIA) launched a programme to develop “gatekeeper” safe AI (link: https://www.aria.org.uk/opportunity-spaces/mathematics-for-safe-ai/safeguarded-ai/), with a specific focus on demonstrating its economic value in real-world applications (TA3). This vision perfectly aligns with the need of power systems, which motivates this project.
Key objectives in this project include:
1. Develop a curriculum of test problems varying in scale and complexity
2. Develop a software platform for training, testing, benchmarking and scaling-up MARL-based solutions
3. Extend the platform capable of ARIA’s gatekeeper AI workflow, and gain application-side requirements to guide the development of gatekeeper AI.
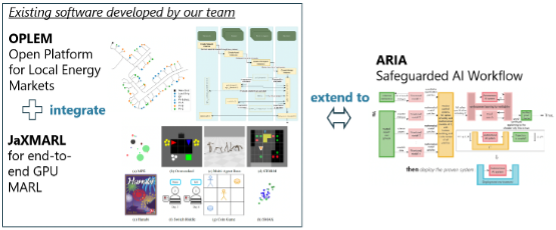
Our Team
Power System Architecture Laboratory (PSAL)
PSAL is focused on power system control and market design.
- Prof. Thomas Morstyn (PI)
- Yihong Zhou (PDRA)
Foerster Lab for AI Research (FLAIR)
FLAIR (https://foersterlab.com/), led by Prof. Jakob Foerster (Co-I), is focused on reinforcement learning (RL), with emphasis on meta-learning, multi-agent and open-ended settings.
Oxford Research Software Engineering (RSE) group
Experience with developing, deploying and scaling up collaborative scientific software.