In-Network ML project: Planter
Planter: Rapid Prototyping of In-Network Machine Learning Inference
In-network machine learning inference provides high throughput and low latency. It is ideally located within the network, power efficient, and improves applications’ performance. Despite its advantages, the bar to in-network machine learning research is high, requiring significant expertise in programmable data planes, in addition to knowledge of machine learning and the application area.
Planter is modular and efficient open-source framework for rapid prototyping of in-network machine learning models across a range of platforms and pipeline architectures. By identifying general mapping methodologies for machine learning algorithms, Planter introduces new machine learning mappings and improves existing ones. It provides users with several example use cases and supports different datasets.
Planter improves machine learning performance compared with previous model-tailored works, while significantly reducing resource consumption and co-existing with network functionality. Planter-supported algorithms run at line rate on unmodified commodity hardware, providing billions of inference decisions per second.
Planter supports targets such as Intel Tofino and Tofino 2, AMD Alveo FPGA, NVIDIA Bluefield-2 DPU, P4Pi (bmv2 and T4P4S), DELL IoT gateway and others.
Contributions by Changgang Zheng, Xinpeng Hong, Riyad Bensoussane, Liam Perreault, Noa Zilberman (Oxford), Mingyuan Zang (DTU), Shay Vargaftik and Yaniv Ben-Itzhak (VMWare).
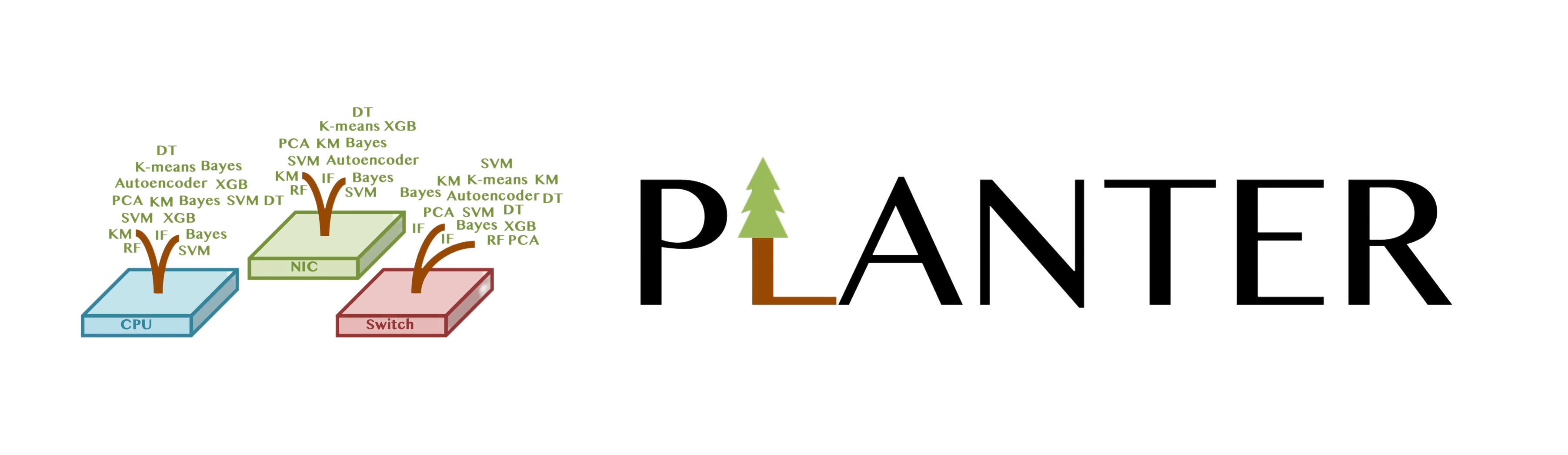