07 Aug 2024
In-Network Machine-Learning paper selected to Best of CCR and presented at ACM SIGCOMM 2024
Paper introduces an open-source framework developed by Computing Infrastructure Group for rapid prototyping of in-network inference on a range of programmable devices such as Raspberry Pi
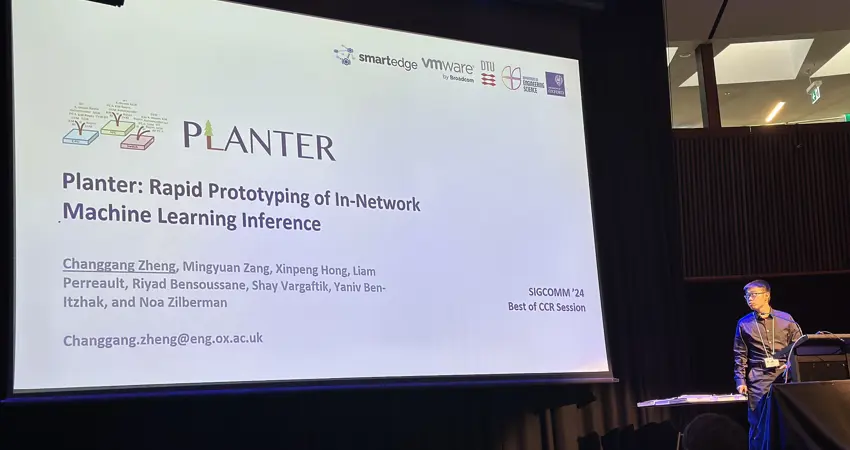
A paper from the Computing Infrastructure Group, ‘Planter: rapid prototyping of in-network machine learning inference’, was selected for ‘Best of CCR’ and presented at ACM SIGCOMM this week - ACM's professional forum for discussing communications and computer networks.
Each year the editors of Computer Communication Review (CCR) select 2-3 papers from all those published in the previous 12 months to be part of the ‘Best of CCR’ session in ACM SIGCOMM, which is the flagship annual conference of the ACM Special Interest Group on Data Communication.
The paper was led by Changgang Zheng, a DPhil student in the Computing Infrastructure Group whose research focuses on developing in-network machine learning solutions. It is co-authored by 7 other authors: Xinpeng Hong (3rd year DPhil student), Liam Perreault (undergraduate student entering his 4th year), Riad Bensoussane (who worked on the project as part of his 4th Year Project and has now completed the MEng), Mingyuan Zang from Denmark Technical University (a visiting student), Shay Vargaftik and Yaniv Ben-Itzhak from VMWare Research (by Broadcom), and Professor Noa Zilberman.
This one-click solution enables researchers to go from a dataset to a real-time deployment within minutes, with little to no prior knowledge.
Their work developed an open-source framework, ‘Planter’, for rapid prototyping of in-network inference on a range of programmable devices, such as network switches (Intel Tofino), FPGA (AMD Alveo), DPU (NVIDIA Bluefield-2), IoT Gateway (DELL Edge Gateway), Raspberry Pi and software switches. This one-click solution enables researchers to go from a dataset to a real-time deployment within minutes, with little to no prior knowledge.
Planter has already been adopted by users in other countries, including Denmark, Norway, USA and Canada, who have applied it to different use cases in the Internet of Things (IoT), e-commerce, smart grid, finance and telecommunications. The EU SmartEdge project uses it in its smart factories and smart junctions use cases.
Professor Zilberman says, “We are delighted that our paper was selected by CCR's editors to this prestigious Best of CCR 2024. We welcome collaborations for follow-up projects to this open-source framework”.
Planter and some of its follow up projects are available at https://github.com/orgs/In-Network-Machine-Learning/.