11 Aug 2023
AI: What Doctors Want and Researchers Need
Oxford study applies AI to predict the future of work, aiming to equip policymakers with better, quantitative information to make evidence-based decisions, shaping this future to the benefit of humanity
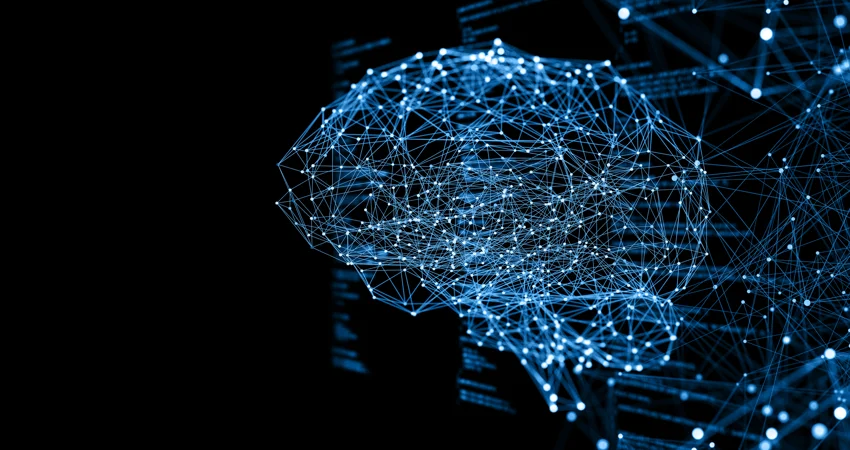
Recent research has uncovered a dramatic shortage of open datasets for AI development in healthcare, especially in areas critically important to medical professionals.
The project leverages Oxford's extensive expertise in applying AI to predict the future of work. It aims to equip policymakers with better, quantitative information to make evidence-based decisions, shaping this future to the benefit of humanity. The new findings highlight the urgent need for more open access to medical datasets, a crucial step that could empower AI researchers worldwide and foster the creation of the AI tools that doctors so urgently need.
"As we move ever closer to a world dominated by intelligent algorithms, it remains vital to understand their impact across society, from finance to healthcare"
In a pioneering Oxford study, Dr. Wolfgang Frühwirt and Dr. Paul Duckworth (2021) employed Bayesian machine learning to better understand the impact the future of work may have on healthcare. Building on the foundational work by their colleagues (Frey & Osborne, 2017; Duckworth et al., 2019), they captured empirical evidence of not only what work could be automated today, but particularly of what should be automated. To this end, the researchers trained AI models on thousands of ratings provided by healthcare professionals.
The results provide the very first detailed quantitative evidence of healthcare practitioners’ preferences regarding the automation of their own work activities. The study identifies numerous specific tasks that are highly automatable using technology readily available today and that healthcare practitioners actually wish to have automated. Interestingly, healthcare professionals desire a surprisingly high proportion of their own work tasks to be fully automated, potentially due to their heavy workloads and occupational health risks.
Precise quantitative estimates of all work activities can be found in the article by Dr. Frühwirt and Dr. Duckworth, ‘Towards better healthcare: What could and should be automated?’, published in a leading journal.
"This study, importantly, recognises that tasks are not necessarily jobs - and understanding how tasks can be automated to the benefit of workers and society seems a vital step in the true integration of AI in primary healthcare.”
Building upon this work, and through a collaborative effort between the Oxford Machine Learning Research Group and the AI Institute at the Medical University of Vienna, another significant step has been taken. The AI researchers analyzed the alignment between current clinical AI research and the practical needs of medical professionals, as suggested in the preceding paper by Frühwirt & Duckworth (2021). They investigated this question specifically in the context of natural language processing, the field of AI that includes large language models such as ChatGPT.
Dr Kathrin Blagec, formerly a Senior Researcher under Associate Professor Matthias Samwald at the AI Institute at the Medical University of Vienna, explains: “We compared the work tasks of a carefully curated catalog of more than 400 datasets and benchmark datasets against the preferences of medical professionals for automation in their daily work activities.”
Dr Frühwirt, who works with the Machine Learning Research Group at the University of Oxford under Professor Stephen Roberts, emphasizes that, “Openly available datasets drive AI progress, but there is a dramatic shortage of open datasets for AI development in healthcare, especially in areas critically important to medical professionals.” He uses AI across various fields to tackle complex real-world problems.
“Openly available datasets drive AI progress, but there is a dramatic shortage of open datasets for AI development in healthcare"
Professor Stephen Roberts, a leading expert and pioneer in the field of AI and applied machine learning, adds, “As we move ever closer to a world dominated by intelligent algorithms, it remains vital to understand their impact across society, from finance to healthcare. We hear much about the potential impact of AI and how it might alter the job market. This study, importantly, recognises that tasks are not necessarily jobs - and understanding how tasks can be automated to the benefit of workers and society seems a vital step in the true integration of AI in primary healthcare.”
The new study has revealed a significant gap between the availability of open datasets for AI development and the clinical and administrative tasks that medical professionals would like to see automated. A previous study (Willis et al. 2019) estimated that 44% of administrative tasks performed in UK primary healthcare can be mostly, or completely automated. Further, the new study did not find a single benchmark dataset that could be used to train AI systems to assist physicians in developing optimal treatment plans for patients or preparing official health documents or records – tasks that medical professionals would strongly prefer to be automated (as per Frühwirt & Duckworth, 2021).
Dr Duckworth, a former Postdoctoral Researcher at the Machine Learning Research Group and the Oxford Robotics Institute, and now a Research Scientist at InstaDeep, states, "Our empirical data highlights a critical gap in AI automation and healthcare work activities. Guiding policy and strategy, this insight underscores the need to align AI datasets with medical professionals' day-to-day tasks. This alignment is vital for AI's continued transformative impact on healthcare, improving both patient outcomes and operational efficiency."
"This insight underscores the need to align AI datasets with medical professionals' day-to-day tasks. This alignment is vital for AI's continued transformative impact on healthcare"
Dr. Kathrin Blagec emphasizes: “The availability of benchmarks that accurately reflect the complexity and diversity of real-world clinical tasks will also be essential for evaluating large language models, advanced AI models capable of understanding and generating human-like text, and assessing their suitability for deployment in the clinical domain.”
The Oxford team hopes their research will catalyze a shift towards prioritizing the creation of open datasets in areas that medical professionals find most relevant to their practice. By better aligning AI capabilities with real-world medical needs, the healthcare sector could witness significant advancements in the automation of key tasks, ones that medical professionals are eager to use. This improvement could potentially lead to streamlined medical processes, enhanced job satisfaction, and ultimately, better patient care.
Envisioning a path forward, Dr. Frühwirt states, “Decentralized approaches, like federated learning, can help to maintain patient privacy while allowing for cross-institutional AI projects. Furthermore, the use of synthetic data, generated by AI trained on real-world data samples, and improved regulation could alleviate the concerns of those hesitant to publish their data."
Further information
The study by Blagec, Kraiger, Frühwirt, and Samwald (2023) was published in the ‘Journal of Biomedical Informatics’: Benchmark datasets driving artificial intelligence development fail to capture the needs of medical professionals
Dr. Wolfgang Frühwirt works with the Machine Learning Research Group (University of Oxford, Department of Engineering Science) under Professor Stephen Roberts. He is the founder and managing partner of object a, a boutique management consultancy firm, specializing in organizational development and AI, as well as a psychotherapist with a focus on executive coaching. Wolfgang enjoys the practical application of AI to tackle real-world problems that would otherwise be too complex to solve. He has led data science projects across multiple domains ranging from improving Alzheimer’s diagnostics through the use of AI to detect subtle changes in brainwaves, to helping companies identify and leverage their biggest AI opportunities using straightforward machine learning methods. His policy is to only accept projects that pose no harm to society or the planet. In addition, he is the lead author of a series of top-tier publications and holds two PhDs, focusing on neuroscience and AI, complemented by a master’s in business.
Dr Paul Duckworth is a former Postdoctoral Researcher at the Machine Learning Research Group with Professor Mike Osborne, and the Oxford Robotics Institute with Professor Nick Hawes, both at the Department of Engineering Science. He is now a Research Scientist at InstaDeep. He received his PhD (2017) from the University of Leeds, working on machine learning for autonomous mobile robots. His current research interests lie in probabilistic machine learning, mobile robotics, and sequential decision making under uncertainty. He is also interested in the impact machine learning technologies have on society and the future of human work.
Professor Stephen Roberts leads the Machine Learning Research Group (Department of Engineering Science), is a Professorial Fellow of Somerville College, founding Director of the EPSRC Centre for Doctoral Training in Autonomous, Intelligent Machines and Systems (AIMS), Director of the Oxford ELLIS unit, a Fellow of the Turing Institute and, until summer 2021, was Director of the Oxford-Man Institute. Stephen is Co-Founder and Scientific Advisor of Mind Foundry and co-lead of Oxford's Schmidt AI in Science Postdoctoral Fellowship programme. Stephen's main area of research lies in machine learning approaches to data analysis. He has particular interests in the development of machine learning theory for problems in time series analysis and dynamical systems. Current research develops a range of methods for diverse problem domains including astrophysics, ecology, finance and data-centric engineering.
Dr Kathrin Blagec is an MD and data scientist and a former member of the Samwald research group at the AI Institute at the Medical University of Vienna, led by associate professor Matthias Samwald. The research group works on strategies for high-impact interventions at the interface of advanced AI, biomedical research, and medical practice. Kathrin specializes in the intersection of healthcare and technology, with a dedicated focus on responsibly harnessing emerging technical tools to optimize their impact on improving the healthcare system and benefiting society. Currently, she works as a data scientist at Symptoma, leveraging natural language processing to enhance clinical decision support for precision medicine.