04 Nov 2021
Performance, safety and adaption: the challenging objectives of modern controllers
Spotlight on work by Xiaonan Lu
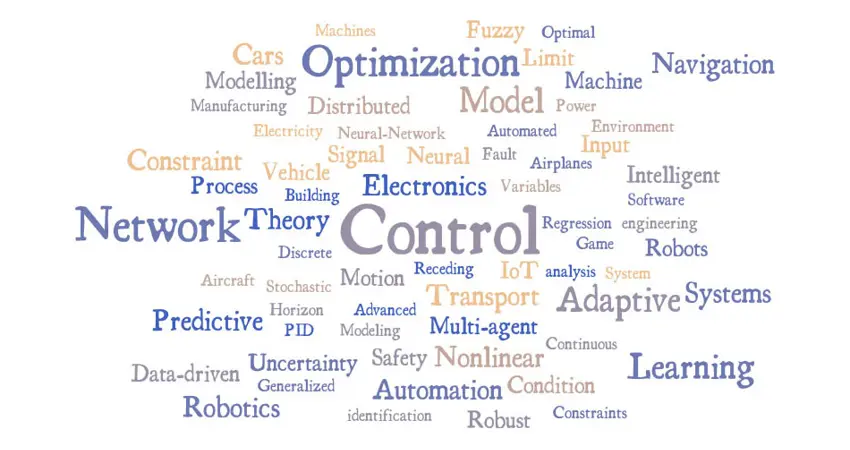
Nowadays, the amount of automation is increasing at a tremendous speed and controllers are required to deal with more complex situations. Although controllers are used in various applications and different scenarios, there are three main themes in the controller design, performance, safety and adaption. Performance refers to the controller’s ability to achieve the desired level or track a pre-set trajectory. Safety talks about satisfying constraints, including state, input and output constraints. Adaption, or online learning, uses the observed data to improve the system model description and enhance performance.
Performance and safety can be achieved using conventional control strategies, such as Model Predictive Control. Model Predictive Control (MPC) approximates the optimal control law and optimize the predicted future performance of the controlled systems based on a model of the system plant. As a result, the performance of MPC relies crucially on the quality of the plant model. On the other hand, adaptive controllers are able to achieve good performance while improving the system description online but fail to guarantee that constraints are satisfied under all possible uncertainties. In short, the input of the controllers has the dual objectives of regulating the system subject to constraints and providing sufficient excitation for the system identification, but they are conflicting in nature.
My research is to propose computationally feasible control strategies that achieve performance, safety and adaption simultaneously. Building on tube MPC, I incorporate the set membership identification method and Persistent Excitation(PE) conditions. The proposed strategies are proven to give satisfactory performance, meet all constraints robustly while reducing the uncertainties associated with model parameters. In particular, simulation shows PE conditions brings a significant improvement in the accuracy of parameter estimation (Left figure) at the expense of an increase in performance cost (right figure).
Left Figure: The volume of the estimated parameter sets using Adaptive MPC with/without the Persistent Excitation (PE) conditions (Shaded area: observed ranges for 30 ensemble disturbance sequences; solid lines: volume averaged over 30 ensemble disturbance sequences). Right Figure: The closed-loop accumulated cost using Adaptive MPC with/without the Persistent Excitation conditions